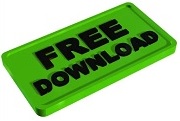
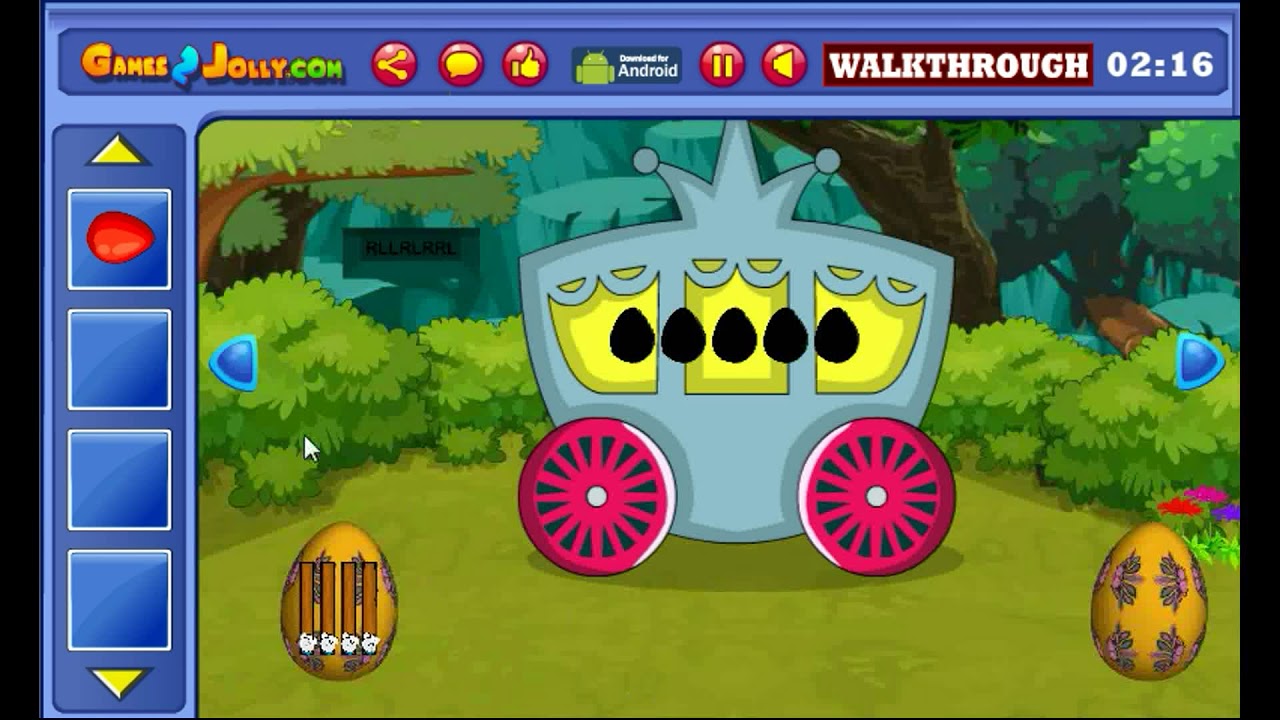
‘Shadow Guard’ appears to be a very well designed early level. So already we see that the first few levels seem to be well designed in terms of keeping interested users’ engagement.
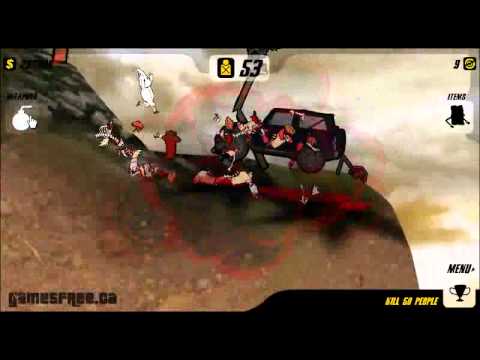
They do account for a lot of absolute exits, but proportionally retain a good amount of their users. By this logic you would expect the first 3 levels to have high exit rates, but in fact level 2 and 3 (‘Gems in the Deep’ and ‘Shadow Guard’) are 13th and 16th for exit rate respectively. You might have guessed from the very high start count that this is the first level, so you would expect it to have the most exits – the game isn’t everyone’s cup of tea. Immediately we see that the ‘Dungeons of Kithgard’ level has both a massive absolute exit count and exit rate. When we sort the Level ratios table by exit rate (ie the proportion of exits to level starts), it looks like this: On a live implementation, this would be easy enough to do. For this demonstration we didn’t dig into cases where users browsed elsewhere in the website before leaving, as there weren’t enough of these cases to make a big difference to the demo. So an exit count of 2.54k for a level means that about 2,500 people left immediately after that level and never came back. When a user plays a level, then leaves the game completely without returning, we defined it as an ‘exit’, and attribute the exit to that level. Let’s have a look at another useful metric: exit rates. Here’s what the filtered ‘Level ratios table’ looks like:įirst off, the value of the first three columns – level starts, level completions and ratio of completion – are good guiding metrics for how the difficulty curve changes between levels, and whether this matches the intended design. There are 21 mandatory levels, along with some optional ones that I haven’t included here for simplicity’s sake. In this game, the first world is free-to-play, after which users hit a paywall to access the next world-stages. To do this, I first filtered the dashboard to only show levels within the first world of the game. Let’s take a look at the ‘Level ratios’ table, and dive into some of the more interesting observations. For product analytics, the most useful of these is the level analytics dashboard, which provides several visualisations of overview statistics to monitor the performance of each game level. The demonstration itself consisted of a number of dashboards we built using Superset, Airbnb’s open source dashboard tool. In an actual client project we can delve into even more detailed aspects of the game, as needed. I was part of the team working on this demo for the GDC and in this post I’ll take you through some aspects of that demo, to show what Snowplow might enable a product manager to do. Our analytics team can also help model the data once it has been processed, to ensure that it is easy to use and in the right format. This is highly granular data about how your users interact with your product and marketing channels. This means that your event data arrive at your database fully usable and fit for purpose.

Codecombat walkthrough a mayhem of munchkins software#
Snowplow Analytics offers a data pipeline management service, using our software to collect, validate, enrich and deduplicate event-level data at source and store it in your data warehouse. Introducing the Snowplow platformĭata can be essential to a product manager’s role, but often the tools that are used are not quite fit for purpose. This example is based on a demonstration of the Snowplow platform built for the CodeCombat coding game, which we prepared for the Game Developers Conference (GDC) in San Francisco. This blog post shows an example of how product managers and game designers can use a well designed dashboard to better understand user behaviour across a game’s levels, design highly playable game levels, A/B test and roll out changes and new features, and ultimately optimise the user experience.
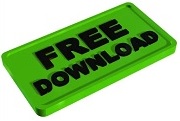